- Data Talent Pulse
- Posts
- π» Making Ethical Business Decisions from Data Insights
π» Making Ethical Business Decisions from Data Insights
DTP #21: How diversity can assist decision making, plus the future of Generative AI
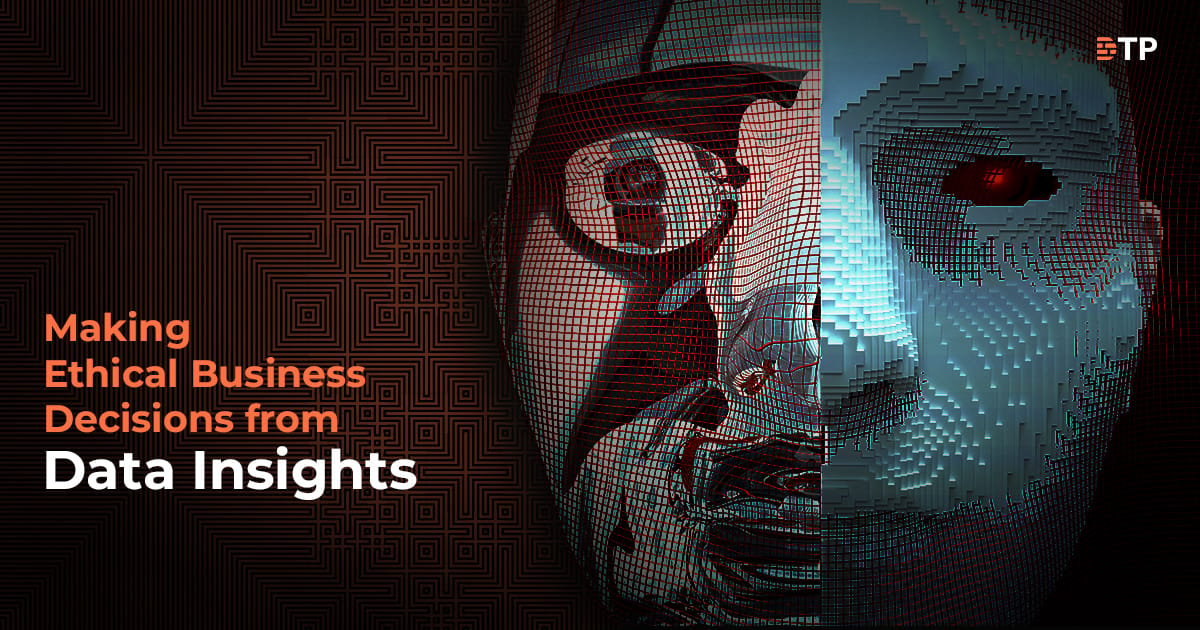
AI-enriched data analytics holds the potential to unlock valuable insights from massive datasets and democratize access to analytics.
Considering this potential, decision makers who have access to these data derived insights have a responsibility to make βgoodβ- i.e., ethical, decisions.
More below.
π From the Web
As AI evolves, it's important to think proactively about ethical implications and ensure technology aligns with human interests. While ChatGPT offers impressive capabilities, we must consider the costs and ethical concerns associated with its use.
The complexity of data ethics often hinders its implementation. Transitioning from principles to an operational data ethics process involves four key steps.
Considering the environmental impact of AI in supply chains is crucial to promoting sustainability. By prioritizing transparency, fairness, privacy, collaboration, and environmental responsibility, ethical AI can enhance supply chain decision-making while reaping its benefits.
Ethically speaking, the ways in which decision makers use insights from data analysis can vary in the spectrum from good to bad.
For example:
A company is using data analytics to identify a correlation between employee attrition rates and marital status, along with working hours. While using data to improve employee well-being and work-life balance is a positive application, the unethical decision arises when the company considers instructing HR to only hire single people based on this correlation, which infringes on personal freedoms.
A decision-making framework, if put in place by a business or an organisation can help eliminate the possibility of making unethical decisions based on data insights.

Ethical Decision Making Framework
A comprehensive ethical decision-making model takes the following into account:
Defining the Ethical Challenge: Clearly articulate the ethical issue or challenge related to data use.
Identifying Stakeholders: Recognize all parties impacted by the decision, including customers, employees, and regulators.
Evaluating Ethical Principles: Consider ethical principles such as transparency, fairness, and privacy.
Reviewing Legal and Regulatory Frameworks: Ensure compliance with data protection laws and regulations.
Generating Options: Brainstorm potential solutions that align with ethical principles and regulations.
Assessing Consequences: Evaluate the potential outcomes, both positive and negative, of each option.
Making an Informed Decision: Select the option that best balances ethical considerations, legal requirements, and business goals.
Implementing and Monitoring: Execute the decision, communicate it to stakeholders, and continually monitor its impact to ensure ethical compliance.
At the start of the process a business leader must identify the specific decision at hand. It is a complex endeavor marked by subjectivity, ambiguity, changing standards, biases, cultural variations, and potential unintended consequences.
Noting the complexity of defining ethical challenges, a diverse team that can bring in varied viewpoints is necessary.
Diversity can enhance decision-making
Workforce diversity and inclusive decision-making are key factors in improving business performance and the bottom line. While companies often emphasize hiring for diversity, it can take years to yield significant results. However, a more immediate approach is to leverage the existing diversity within the organization by incorporating diverse employees into decision-making processes at all levels.
Research conducted over two years, analyzing 600 business decisions made by 200 different teams in various companies, revealed compelling insights:
Inclusive Teams Excel: Inclusive teams make better business decisions up to 87% of the time.
Speed and Efficiency: Teams that follow an inclusive decision-making process make decisions twice as fast and reduce the number of meetings by half.
Better Results: Decisions made and executed by diverse teams deliver 60% better results compared to non-diverse teams.
These findings underscore the importance of aligning diversity and inclusion initiatives to drive optimal business outcomes. A diverse workforce contributes to better decision-making, with decision quality improving as team diversity increases. Gender-diverse teams make better decisions 73% of the time, whereas all-male teams do so 58% of the time. When teams also encompass a range of ages and geographic locations, they make better decisions an impressive 87% of the time.
Unfortunately, non-inclusive decision-making practices remain widespread, with all-male teams responsible for roughly 38% of decisions in typical large companies. The situation is even direr in less diverse sectors, like the technology industry, where teams with women participate in less than half of business decisions.
Recognizing this challenge, inclusive decision-making presents a significant opportunity for companies to enhance their business performance. Improving decision-making processes can offer a substantial boost to a company's success.
π» Platform/Tool Highlight
360ofme: A personal data exchange platform provider enabling the secure storage, sharing, and monetization of data between businesses and consumers.
ODI Maturity Model: A way to assess how well an organisation publishes and consumes open data and identifies actions for improvement.
Ethical Data Science Framework: A comprehensive framework to manage ethical concerns in data science projects.
πΌ AI in Business
Is Generative AI A Bubble?

An article on KDnuggets speculates that unsustainable hype and limitations of current technology will burst the Generative AI bubble:
It draws a parallel between the current generative AI hype and the late 90s dot-com bubble.
The dot-com bubble burst due to flimsy business models, lack of profitability, and overreliance on investor funding.
Generative AI's rapid growth and hype have similarities to economic bubbles, with expectations possibly exceeding reality.
The article highlights limitations in today's Generative AI, such as low-quality outputs and scalability challenges.
It suggests that valuations of generative startups may be unrealistic, leading to a loss of funding and declining stock prices.
Concerns surrounding the Generative AI bubble include a slowdown in adoption, high capital requirements, economic factors, and legal and ethical concerns.
To mitigate the risks, the article suggests focusing on practical business use cases and not relying solely on hype and demos.
The future of Generative AI remains uncertain, with the industry's sustainability and long-term success in question.
π€ Prompt of the week
You are a data analyst. I have a dataset of [Description]. Write python code to clean the data by removing missing values, duplicates, and outliers.
[Description]: Describe the elements of the dataset. Ex. Order ID, customer ID, order date, product ID, and quantity.
See you next week,
Mukundan
Do you have a unique perspective on developing and managing data science and AI talent? We want to hear from you! Reach out to us by replying to this email.
Reply