- Data Talent Pulse
- Posts
- 👩💻 AI Related Data Privacy Within Businesses
👩💻 AI Related Data Privacy Within Businesses
DTP #26: Q&A with Antonio Rocha

We spoke to Antonio Rocha, Data Privacy and Protection Expert, and Data Leader, on how AI is affecting data privacy, the differentiator between typical software startups and AI startups, and steps governments and businesses can take to ensure data security.
Here’s a quick summary of the Q&A below:
Data as it relates to startup innovation and AI
Building for a problem, and building a product
Keeping users in mind when utilizing data
Governments and data security
First steps towards protecting and utilizing sensitive data
Quotations lightly edited for concision and readability.
🌐 From the Web
Under GDPR, controllers must disclose personal data processing details in privacy notices. When utilizing personal data for AI training, disclosures should specify lawful purposes, data recipients, data transfers, retention periods, access/rectification/erasure rights, and opt-out options.
Emerging laws aim to uphold responsible AI use, emphasizing individual data control in automated decision-making. Companies integrating AI must comply with evolving regulations, as seen in the EU's risk-based AI Act and GDPR, and various state-level U.S. laws, including California's CPRA. Compliance involves assessing and adhering to specific data protection requirements.
Data privacy expert Brittany Kaiser cautions against inadequate regulation of tech, advocating prioritizing data privacy protection over hastily regulating AI without comprehensive definitions and federal data laws.
On AI, do you find that it's changed the privacy domain?
In so many ways. So my background first, to give a little bit of perspective or context. Before I went into the data space as a technical and business field, I had around 10 years of experience with big corps, major telecom, banks. I was always dealing with lots of customer information and information systems, but that somehow always missed the insights realization.
Meanwhile, in 2008 everyone in banking was hit, but going through many US information pieces while I worked in banking doing credit analysis, I was able to realize the seeds of the crash were planted much before, and that anyone with inside trade knowledge could have kind of predicted where things were going; but always lacked the “big data” for mathematical proof. So around 2010 I decided to get into startups and help a few founders and become one myself. I was one of, let's say 100 people or more, who helped kick start the Lisbon startup ecosystem. There was a lot that I could offer, especially in the FinTech space, but I always knew these startups had to be very techy to be able to cut through the noise of much larger vendors. At that time, AI was just emerging; as companies started to tap into their datasets and apply to data more unconstrained ways to extract insights. Once the ecosystem was launched and stable, it was time for me to move elsewhere; I needed a much larger pond and decided to move to London.
There, when I was working for Software AG, I spotted an opportunity to open an AI firm, and along with other people, we did that here in London & Cambridge.
That was when we really started to do some interesting work; i.e. our first project was a risk assessment algorithm to classify maritime assets in the Mediterranean; and allowed some depth of insights due to the combination/manipulation of some data to extract possible behavioral patterns in very large datasets.
At that time, as an AI Startup studio/practice that wanted to build AI engines focused on particular problems, and then launch an AI VC fund, we were always looking for new things to do with data that involved AI. But it was a pretty raw environment. We had cofounders with pens in their pockets with 1,000,000 customer records walking around the city, so as you can imagine it was pretty raw, right.
There's really very little in life that resembles a startup. It’s a bit like doing an MBA in 6 months, in real life, while you’re setting up a real business and going “backwards” in the feedback loop every single day. It’s a tough journey; but the learning can't be taken away from you, ever. At the time, I realised, wait a minute, we’re kind of on the brink of a startup, data, AI revolution because, well, once you have 1,000,000 customer records in a pen, in a pocket of someone who is not even out of college, you are on the brink of something new. When you add on top the ability to apply a model, that is better in accuracy and speed to anything a big company has, that’s another layer, and so on. Layers accumulate and produce a wave.
On a broader scale, do you think there's a trend of AI startups and founders being careless about where they get their data and how they use their data?
There has always been some degree of experimentation and dirtiness in innovation. While this is looked upon as “bad” by some in the ecosystem; what most kind of miss is that in data science and lean startups, we’re just applying the scientific method (that requires hypothesis/question-proof, combined with layer upon layer of insights. For context, my startup started when we won an EU funded innovation contest, basically matching big corps with innovators. Anything of value passes through innovation phases, and the first ones are dirtier, so they require a looser mesh of controls, or ones that are more adaptative/flexible. It’s product development 101.
So, at the same time, while I could go easy into “oh Startups are really bad.” not necessarily; and very much the contrary! It's by the event of innovation that we do something new, that we expand the knowledge of the world and that we create things out of nothing. And that process is in itself radical, and radical is a little bit dirty. Otherwise, it's not valuable, usually. Only by luck, and as any mathematician will tell you, luck is a rare event by simple understanding.
It is necessary to be a little bit radical to say the least, to create innovative solutions that end up bringing whole new classes of products [and] of ways of thinking, of ways of usage and I'm pretty sure that [the] Chat GPT class, so to speak, is barely starting. They have, in a very short amount of time, evolved tremendously. One should not jump easily into oversimplifications of complex events with many dimensions.
That makes sense. Do you think while people are trying to innovate, there are still steps that they can take to do it in an ethical way?
Certainly, and I'm going back to my own experience - back in the day we had this situation where one government asked us to build an AI to help find children at risk of being molested. This was back in 2015. In a time when nobody was even talking about this, let alone AI Ethics! We had to make some pretty harsh product decisions, not only to secure the children, but also to look at exploitation and possible future risk.
We could have easily developed this product, but products need to be developed with the right people, for the right reasons, and very close to users or their targets. We ended up delivering a product that added a little bit of information vs. one that included lots of AI but could also easily go off a cliff and do some real damage. Due to the fact that we were very far away from the software users, and very far away from the humans who would be impacted by this, we said to ourselves, well, we're just going to deliver this little bit of feature here.
And if anyone wants more? They will have to come back. And we're going to have to strengthen the controls around the way the information is used. We did this because we quickly understood the rest of the ecosystem (partners, users, etc.) wasn't mature enough. At best, there was bare bones understanding of the tech, its need to be updated, changed, re-evaluated, etc. It’s a bit like handing a gun to a small child. We could have facilitated the product to a degree that some of the risks wouldn't be there and that probably the users would [have] more features, but once we were making this design decision, we were thinking about protecting the humans that this decision would affect.
But yeah, it's tricky because you really need to develop a product from a perspective of how much impact will this have and how this may affect a human in the end of this product, that is not even interacting with the product.
So we thought about it from an information impact point of view and how this information might affect this person or not and what is useful in terms of the world to receive about this particular person in terms of protection. Those were the design questions that helped us.
Would you say most people think about those questions in advance or is it once they start to work on it that they get the chance to?
Data science software is a discovery process, vs. a normal software product point of view which is fairly mapped. Even normal software goes through a strong discovery process from requirements to design choices, a plethora of choices, each with its own impact downstream. [From the] development perspective of AI, we have to think back about the scientific process, the scientific reasoning, and we need to find data to prove, improve or find something totally new or ways to solve small problems at scale with less constrained inputs
So I was curious about that side of things where governments are trying to take advantage of emerging tech and the precautions that they're taking, or the lack thereof.
Yeah, that's definitely a tricky one! Well, today we live in an age where It's fair to say that governments are still not educated from an information management choices point of view and governments systematically have had a need to control. You know, the whole premise of government is to control something for the wider public.
If we look into the evolution of laws, the evolution of the use of data in time, we can see a stream of events where there is in the last 5 years an increase in the usage of data to take away certain freedoms.
Most states have been using data to control citizens in various aspects of their life’s, from accessing a government issued document to knowing where the citizen is travelling, to what internet searches are done, particular keywords that prompt certain actions and special buckets, to even control what a citizen can say online.
It’s fair to say that a large majority of the human population is already under varying degrees of AI control; from this point of view.
The fact that the majority of these algorithms don't go through any sort of visibility, awareness, accountability, public and/or societal input and governance; should be enough reason to worry everyone. We don't even have in place enough governance to detect the little errors; let alone widespread outputs that escape our siloed, information swamped minds.
I would definitely prefer to see humanity use the skill of information management and software management, in ways that empower us to use information better. From a government perspective, I would definitely prefer to see citizens having much more access to data to improve the work of government through transparency, because governments exist for citizens, they don't exist as an entity that is supposed to control them, in my view. Open data and data ecosystems are very interesting tools that could promote that.
What initial steps can anyone, whether it's a government or business, take to ensure that they are properly utilising data, for data security and to protect sensitive information?
This is a very complex subject to get into [in just this small interview], but I can say a few things that will make an impact.
So, I would say the first and foremost one of the biggest things that I've experienced, is always lack of collaboration and understanding. What usually happens in companies, governments, etc. is that you have a combination of people going into a problem from their own siloed view, so you've got the security guy, you've got the databases guy, you've got the, the program manager, businessperson, stakeholder, etc.
So, we can multiply this for at least 10 or 15 people. And they're always lacking collaboration in the sense that they attach themselves to a project and deliver their own point of view, but they're not very concerned with the depth of that point of view or the implications/dimensions of a lot of the design choices. There’s no depth, no governance, no way to get a flag back, “something is not right”. We humans manage complexity very badly. There's a combination of outputs, so as specialists our job is to build a governance forum where we discuss these things. We go over the design, we understand the implications, we do tests, and we find solutions and bridges in terms of the knowledge that we share with each other, that allow the group to have [an] understanding and respect for the implications of the stuff that we're building. It's as simple as that. It obviously takes time and effort, and it must be added to cost too.
The thing is, we need to change our thinking because 20-30 years ago the industrial silos, skills, jobs, were so divided that the implications of our work could only be seen way, way further ahead. Now we are reaching a state which I would describe as a state of data fusion throughout the world; where we have capabilities to mix and match everything to such a degree that immediately we can build immensely powerful data products, while at the same time, the implications of those products can also be exceptionally bad. Unfortunately, due to poor complexity management skills, lack of governance and controls, it’s very easy for this to happen and shape the experience of humans negatively; from small impact experiences to terminal ones. The more we speed up and lose complexity management and governance skills; the more we augment the risk of having to be taken over by a machine, that will one day break also, inevitably. I don't think that would be smart of us. I think we can do better than that. I think we have more skills than that.
💻 Platform Highlight
RSA Archer: An integrated risk management / GRC platform from RSA Security.
SAI360: A cloud based EHS and GRC platform from SAI Global
Piwik PRO: A privacy-oriented alternative to Google Analytics.
💼 AI in Business
AI and Data Privacy – Top Tips
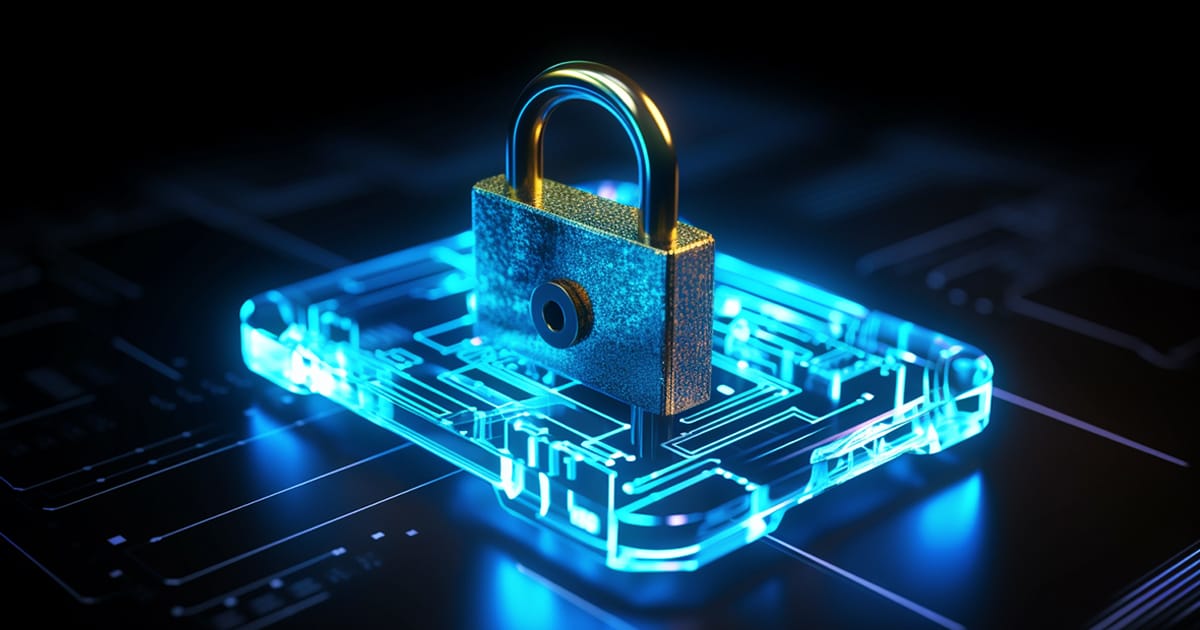
Image generated by Midjourney
An article from Forbes discusses privacy concerns with AI. Generative AI, such as ChatGPT, is rapidly gaining popularity, but businesses should be aware of the potential data privacy and ownership implications before using these tools.
Key Points:
Generative AI tools like ChatGPT do not guarantee data privacy.
ChatGPT collects IP addresses, browser types and settings, and uses cookies to track browsing activity.
Chatbots may not be able to fully comply with data privacy regulations like GDPR.
Businesses could be held liable for any violations resulting from their use of generative AI.
Recommendations:
Businesses should vet generative AI services like they would any other vendor.
Ensure you understand the service's data collection and privacy practices.
Develop a training plan for employees on how to use generative AI responsibly.
Proactively address privacy concerns to mitigate risks and establish yourself as a privacy leader.
👨💻 Word From Our Data Scientists

💬 Social Highlight
Reddit - Should the "data" part and "scientist" part be two separate jobs?: Post
"GRC and how It helps with Data Privacy” - A thread
🤖 Prompt of the week
Can you suggest some ways to protect my data while using ChatGPT?
See you next week,
Mukundan
Reply